Big Data World – Learn, Unlearn, Relearn
People moving on from the traditional data world to the big data world need to learn new concepts, unlearn old irrelvant stuff and relearn some concepts in the new light of big data. This post is about creating a mindset to learn about big data.
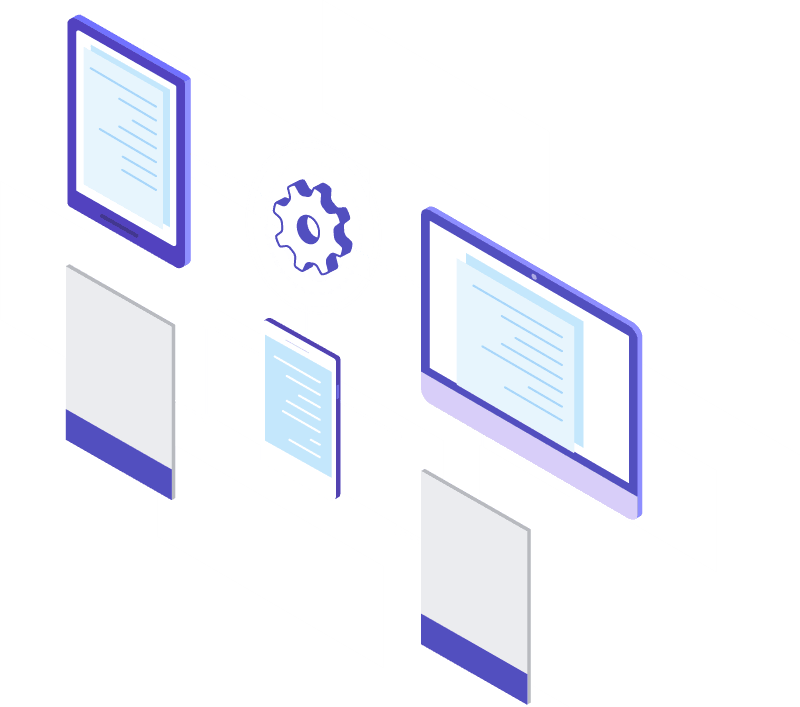
Everything is data
The data we used to work with decades ago was small, exact and causal in nature. Most of the times the data we used for analysis would fit into a spreadsheet. It may have sampling and randomization in it. We were conditioned to use only structured data. We see only structured data as data and everything else as junk. We need to unlearn this and embrace that everything is data. If you look at big tech companies like Google, Facebook, Twitter and the data they collect about their users from various products you would understand that everything is treated as data. Image, frame inside video, location, speed, direction, biometric like Iris recognition, facial recognition are also data. The first step in bringing a data-driven culture into your organization is to embrace this mindset that everything is data and then work on a datafication project which defines how to collect, standardize and quantify the data.
Causal Analysis
Data was used to understand the past as deeply as we can. We used data for root cause analysis using various techniques like 5 Whys and Pareto Diagram. There are two ways in which we arrived at conclusion about the past. One is deductive inference and the other one was inductive reasoning. An example for deductive inference would be that females of mammals are milk feeding and not egg laying. Of course there is always an exception and if you find that exception you can post it in the comments. That helped people classify goat, cow, ape as mammals. The other method we use to come to conclusion about past was inductive reasoning. Evolution, Climate Change, Pandemic, Pangea, Big Bang Theory. In inductive reasoning we conclude without the existence of actual object to be examined but based on similar objects that we examined. Even today inductive reasoning is used in many industries in their quality control department which presents statistics to their management. They use sampling with randomization to guess the quality. We need to unlearn this concept and embrace a new one in the big data world.
Andon cord
Andon cord is a technique or process that is used in Japanese automobile companies where workers in assembly line will pull a cord when they notice any issue in the assembly line and stop the production and bring it to their managements attention. We need to rethink all the processes in the companies in the light of Andon cord concept. Imagine in a manufacturing firm where the product goes through various sections of the company. eg: in apparel manufacturing the raw material fabric goes thru dyeing, compacting, cutting, stitching, quality check and ironing and packing. Mistakes can happen in any stage and we need to collect data at each stage and develop a digital Andon cord that will analyze the data real-time from all these sections and alert the management about potential issues and catch the defects at very early in the manufacturing cycle at an individual item level. Previously this was not technically feasible now with IoT, computer vision this is now very much feasible and is a reality. This should convey what mindset people should have when they drive big data projects.
Data Then vs Data Now
Data was used in the past to understand the past and keep track of the history. Now data is used to predict the future. Data had a very small lifecycle and it was mostly used for Year over Year, Month over Month, Week over Week comparisons and then shelved. Now data has a perennial use and it will be used to solve various problems in the enterprise. Data was used for causal analysis but now it is used for predictive analytics. Data was accurate at micro level but in big data world we compromise accuracy and we focus on the insights more than accuracy.
Correlation
Correlation replaces causal analysis in the big data world. Correlation is a way to define the statistical relationship between two values. Correlation is used for prediction. One good example of correlation is from ecommerce. Let us assume you own an online electronic store that sells tablets. Looking at the sales data for the tablets you can infer that for every 100 tablets sold 60 customers bought screen protector and 30 customers bought cover. Now you can find a quantifiable relationship between tablet sales and screen protector sales, tablet sales and cover sales. To predict the sales of screen protectors you need the input of table sales. Now this is the biggest advantage of correlation in the big data world. The challenge here is the sometime correlation can be between totally unrelated products like tissue paper and sanitizer during the onset of corona crisis. This is where machine learning helps.
Conclusion
Hope this post explain the mindset that organizations need to embrace before diving into the big data world. This is what Knowillence is specialized on. Knowillence helps companies redesign their processes in the light of big data and help them build modern infrastructure that empowers everyone in their organization. Reach out to us if you need any help in the big data area.
H.Thirukkumaran
Founder & CEO
H.Thirukkumaran has over 20 years of experience in the IT industry. He worked in US for over 13 years for leading companies in various sectors like retail and ecommerce, investment banking, stock market, automobile and real estate He is the author of the book Learning Google BigQuery which explains how to build big data systems using Google BigQuery. He holds a masters in blockchain from Zigurat Innovation and Technology Business School from Barcelona Spain. He is also the India chapter lead for the Global Blockchain Initiative a non-profit from Germany that provides free education on blockchain. He currently lives in Chennai India.